Segmed’s Role in WHO Framework for AI Medical Devices

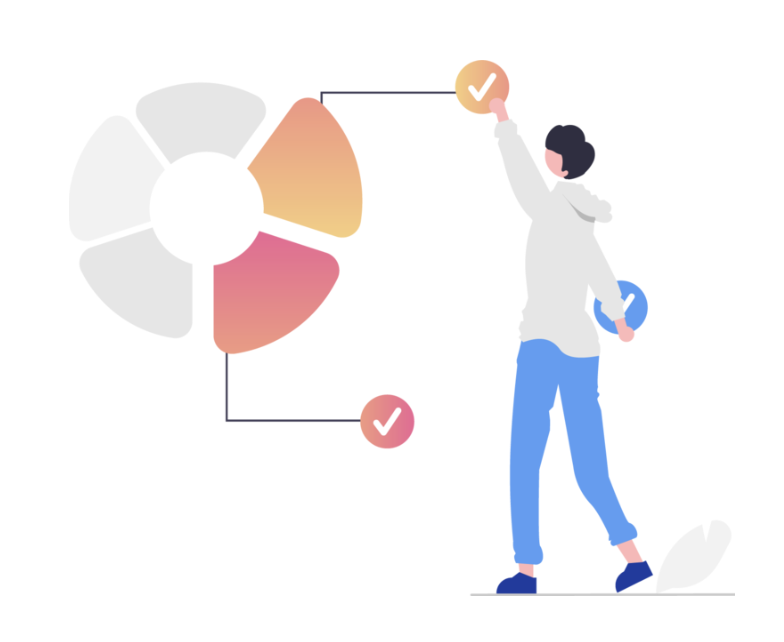
Developing an AI algorithm that will make it into the clinical setting is not easy. The whole process is challenging, from gathering and curating medical data, to training, validating, and testing an AI model, and finally to deploying the algorithm in the clinics. The World Health Organization (WHO) recently published a framework that helps developers of AI-based software as a medical device (AI-SaMD) to understand the requirements of evidence generation from development to post-market surveillance [1].
It is expected that AI will significantly improve healthcare delivery and outcomes. The clinical application of AI models is, however, still challenging, not only in low- and middle-income countries, but also in high-income countries. One of the biggest hurdles for clinical application of AI algorithms is access to well-curated and diverse data used for training, validation, and testing. Many AI models are developed in an academic setting, based on homogeneous data from a single or a few academic institutions. Scientific papers often show excellent validation results of these algorithms, while performance in real world clinical settings is limited. A framework to guide AI developers in this process, is thus important.
The WHO framework divides the process for medical AI model development into three steps:
- Software development
- Software validation and reporting
- Deployment and post-market surveillance
The first step (software development) includes how to manage and curate data, how to determine the ground truth, and how to train and internally validate the model. The next step (software validation and reporting) includes external validation and how to report the evidence. And the last step (deployment and post-market surveillance) includes evaluation of clinical usability and clinical impact of the AI model.
The WHO describes standards of data handling and ground truth determination based on a paper published by the Segmed team in collaboration with scientists and physicians from Stanford University, the National Institutes of Health, and Hardian Health [2]. In this paper, we described the data management process ranging from data access, selection, de-identification, to labeling. All of these data handling steps are part of the Segmed process.
The WHO describes standards of data handling and ground truth determination based on a paper published by the Segmed team in collaboration with scientists and physicians from Stanford University, the National Institutes of Health, and Hardian Health.
The recently published WHO document offers an excellent framework to how evidence of AI-based medical devices can be generated. Adhering to this guideline, however, is not straightforward, since large amounts of heterogeneous and standardized data are needed. Over the last 3 years, the Segmed team has worked on solving this problem. AI developers who want to develop an AI model that will be applied in the clinical setting can now build tailored, anonymized, well-curated datasets through the Openda platform. Most importantly, currently the data acquisition time is generally at least 9 months, but oftentimes multiple years. With Segmed Insight this can be shortened to 2 weeks. And since the data is also originating from non-academic hospitals and clinics in different geographic areas, the generalizability of the AI models is expected to be much better, resulting in less biased algorithms.
In short, the new WHO framework helps AI developers with evidence generation of clinical AI algorithms. And the data collection process can be simplified and sped up with Segmed Openda.
References:
[1] World Health Organization, Digital Health and Innovation Team, Medical Devices and Diagnostics. Generating Evidence for Artificial Intelligence Based Medical Devices: A Framework for Training Validation and Evaluation. ISBN: 978-92-4-003846-2. November 17, 2021.
[2] Willemink MJ, Koszek WA, Hardell C, Wu J, Fleischmann D, Harvey H, et al. Preparing Medical Imaging Data for Machine Learning. Radiology. 2020 Apr;295(1):4–15.